A Radar-Nearest-Neighbor Based Data-driven Approach for Crowd Simulation
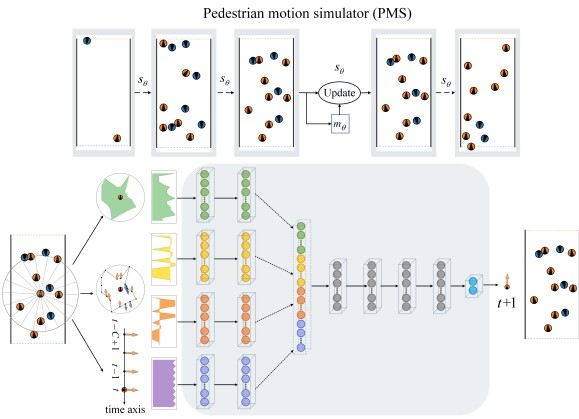
Problem & Motivation
1.Crowd simulation is an effective technology for the response in public emergencies. Scholars have developed many knowledge-based models to mimic human behaviors by converting expert knowledge into dynamic models. While the introduced hypothetical rules in models cause a certain degree of difference from reality.
2.With the development of artificial intelligence, data-driven methods have been increasingly applied to the simulation of crowds. However, the application of AI in crowd simulation is still in its infancy. Relevant considerations and treatments for modeling the interactions of pedestrians in these data-driven methods are relatively rough and need to explore deeply.
3.To overcome these shortcomings, we develop a novel Radar-Nearest-Neighbor (Radar-NN) method and establish a data-driven crowd simulation model based on the proposed Multi-Feature Fusion Recursive Neural Network (MFF-RNN).
Methods
1. We develop a novel Radar-Nearest-Neighbor (Radar-NN) method by treating the studied pedestrian as a radar to detect its surrounding environment within a receptive field. This method is more comprehensive than the k-Nearest-Neighbor (kNN) (Ma et al., 2010) method, and can acquire the space around pedestrians in all-around ways.
2. A novel and thorough motion states of pedestrians are designed based on the developed Radar-NN method, which achieves more comprehensive feature expression. Besides, the movement inertia of pedestrians is considered in the motion state and represented by the previous velocity sequences.
3. To fuse the input features with different attributes and find the mapping from the motion states to the velocities, a Multi-Feature Fusion Recursive Neural Network (MFF-RNN) is established.
4. Five evaluation metrics are proposed to quantitatively assess the performance of the approach.
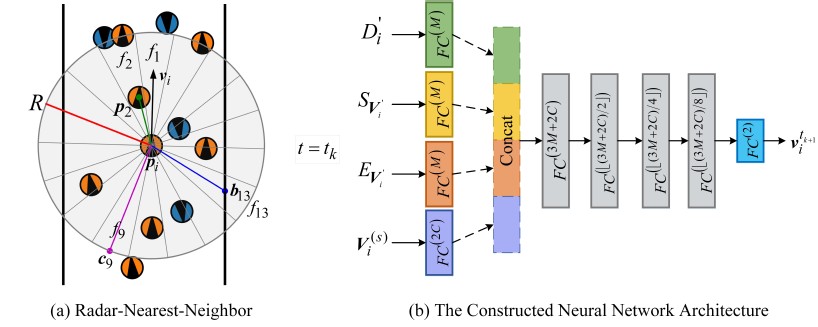
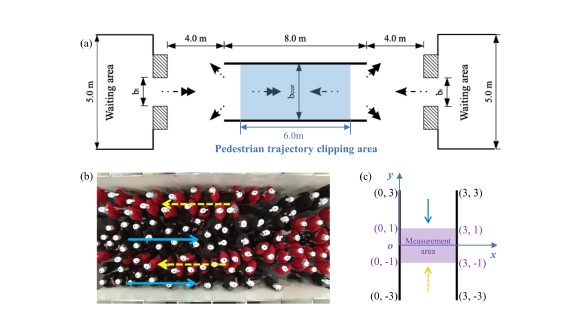
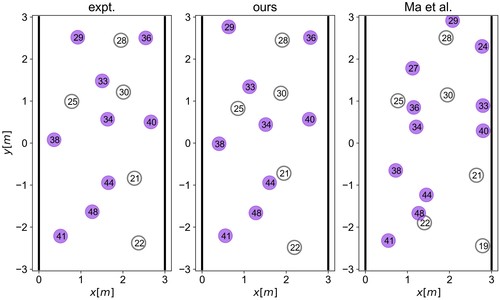
Related Publication
Zhao X , Zhang J , Song W . A Radar-Nearest-Neighbor based data-driven approach for crowd simulation[J]. Transportation Research Part C: Emerging Technologies, 129.
Download: [Paper]