Artificial neural network based modeling on unidirectional and bidirectional pedestrian flow at straight corridors
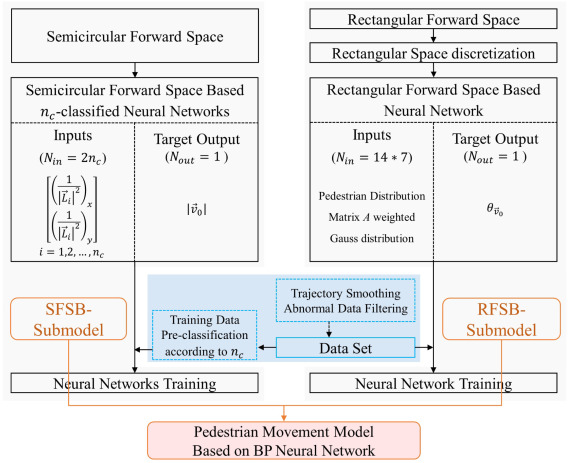
Problem & Motivation
Pedestrian movement modeling is a primary and effective method to simulate and predict pedestrian movement. Lots of pedestrian movement models have been built and presented many significant phenomena. However, there still remains some problems and challenges. For instance, the modeling process is relatively complex because of various mathematical formulas and movement rules. It is difficult to take individual differences of pedestrians and other complex influence factors into account. Furthermore, the parameters in most of pedestrian movement models are adjusted using small part of experimental data, which makes the generalization ability of model poor.
The rapid development of Machine Learning brings us great hope to overcome difficulties. We propose an artificial neural network based model to mimic pedestrian behaviors in two typical and common scenarios (unidirectional and bidirectional flow).
Methods
In the proposed model, the pedestrian velocity vectors are predicted with two sub models, Semicircular Forward Space Based sub model (SFSB-sub model) and Rectangular Forward Space Based sub model (RFSB-sub model), respectively. Both unidirectional and bidirectional pedestrian flow at straight corridors are investigated by comparing the simulation and the corresponding experimental results from several different aspects (pedestrian trajectories, fundamental diagrams and quantitative trajectory error).
Unidirectional flow
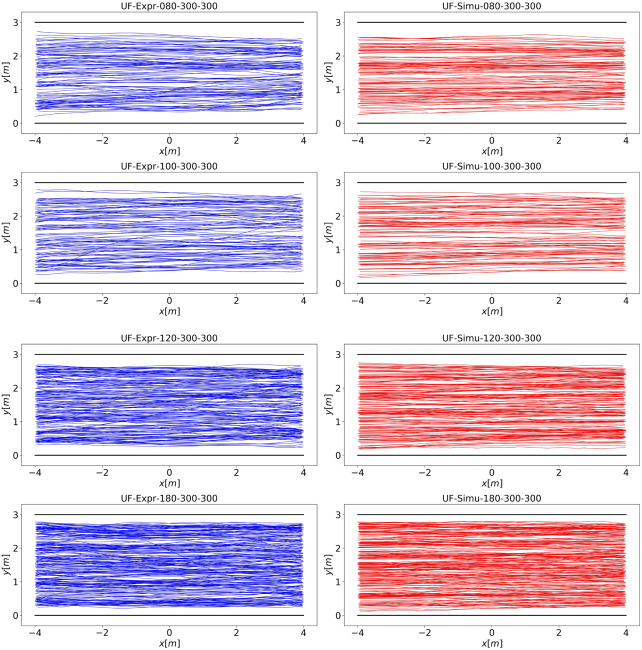
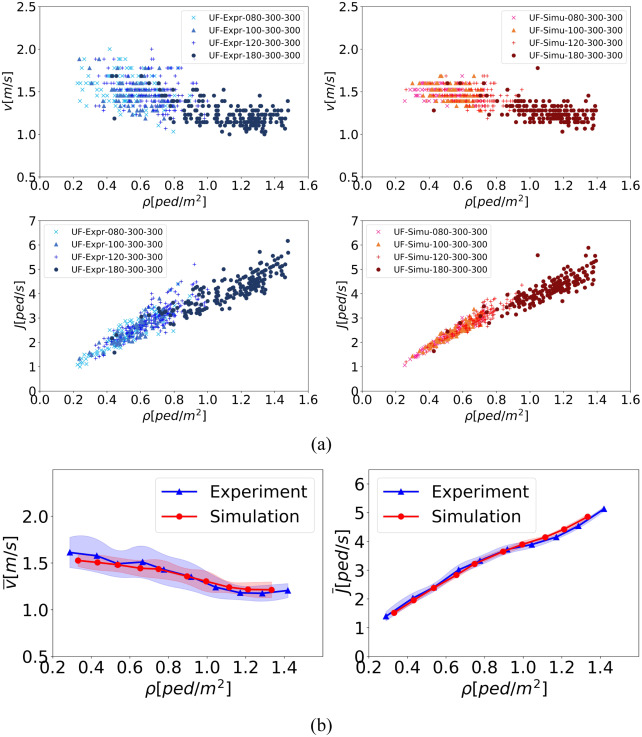
Bidirectional flow
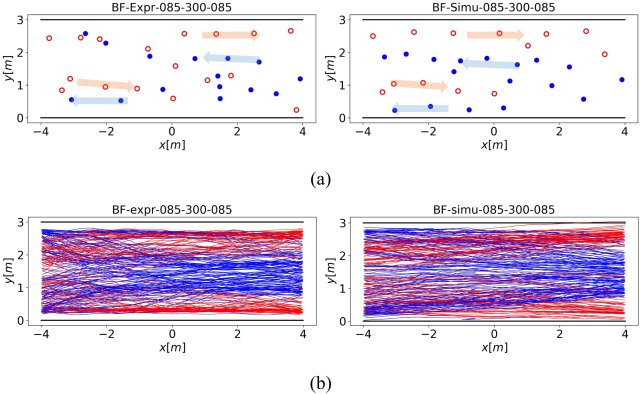
Related Publication
Zhao, Xuedan, et al. "Artificial neural network based modeling on unidirectional and bidirectional pedestrian flow at straight corridors." Physica A-statistical Mechanics and Its Applications (2019).
Download: [
1-s2.0-S0378437119321272-main.pdf] [Link]